

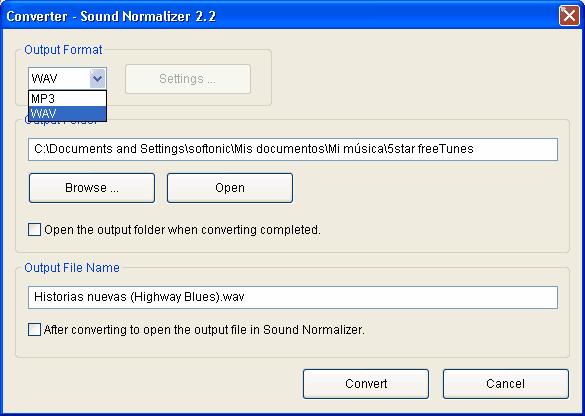
8(3), 643–674 (1996)Īrpit, D., Zhou, Y., Kota, B.U., Govindaraju, V.: Normalization propagation: a parametric technique for removing internal covariate shift in deep networks. 173–182 (2016)Īn, G.: The effects of adding noise during backpropagation training on a generalization performance. In: International Conference on Machine Learning, pp. KeywordsĪmodei, D., et al.: Deep speech 2: end-to-end speech recognition in English and Mandarin. As a result, MBN works very well even when the batch size is very small (e.g., 2), which is hard to achieve by traditional BN. With a dynamic momentum parameter, we can automatically control the noise level in the training process. To reduce the dependency of BN on batch size, we propose a momentum BN (MBN) scheme by averaging the mean and variance of current mini-batch with the historical means and variances. Since the regularization strength of BN is determined by the batch size, a small batch size may cause the under-fitting problem, resulting in a less effective model. Such a noise generation mechanism of BN regularizes the training process, and we present an explicit regularizer formulation of BN. To make a deeper understanding of BN, in this work we prove that BN actually introduces a certain level of noise into the sample mean and variance during the training process, while the noise level depends only on the batch size. The success of BN has been explained from different views, such as reducing internal covariate shift, allowing the use of large learning rate, smoothing optimization landscape, etc. As one of the most popular normalization techniques, batch normalization (BN) has shown its effectiveness in accelerating the model training speed and improving model generalization capability. Normalization layers play an important role in deep network training.
